Why Your OTT Platform Needs a Recommendation Engine?
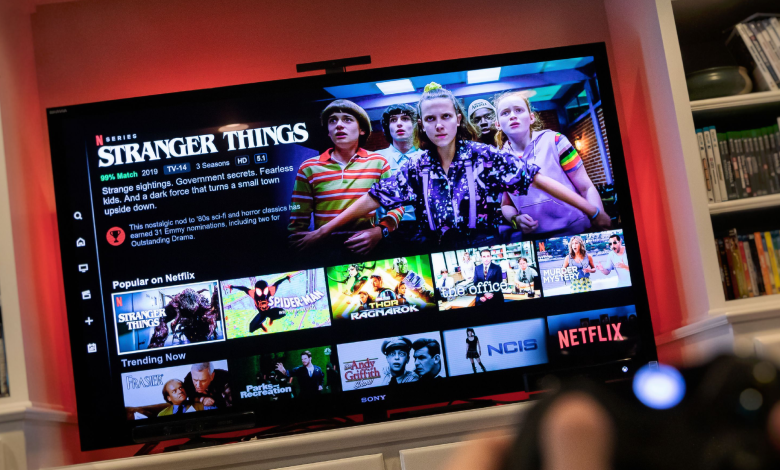
With the rise in the use of web services like Amazon, YouTube, Spotify, Myntra, etc. recommendation systems have assumed an important part in our lives. Recommendation engines are algorithms that are designed to recommend the relevant subject matter to the users of an app.
In e-commerce, online advertising, and OTT video platform, recommendation engines play a very crucial role. They can generate enormous revenues and at the same time make you stand out considerably from your competitors.
OTTs are competing to satisfy viewers’ behavioral shifts and preferences of users across different geographics. This requires the use of data-driven knowledge and Artificial Intelligence. Therefore, recommendation engines for OTTs execute and modify content delivery and analytics for better user engagement.
Here is a small statistic to let you know how indispensable recommendation engines are for revenue-making in OTT platforms. In the entire OTT Video segment, the largest segment is OTT Video Advertising with a market volume of US$180.40bn in 2022.
To make ads valuable and targeted on OTT platforms, sponsors rely upon facts and statistics assembled by recommenders. This blog walks you through the importance of recommender systems for OTT platforms in detail.
What is a Recommendation Engine?
A recommendation engine is a system that uses machine learning algorithms to collect, store, filter, and analyze data which is based on customers’ ratings, preferences and search history, etc. It functions to recommend the most relevant content and product suggestions to the user, using its capabilities to work with the data collected.
It helps viewers of OTT platforms save a lot of browsing time, as it directs the user to the content that he will presumably like. E.g. If a user likes to watch ‘Mission Impossible’, then he gets recommendations for movies of the action genre and the movies in which Tom Cruise is in the cast.
OTT platforms save a lot of browsing time, as it directs the user to the content that he will presumably like. E.g. If a user likes to watch ‘Mission Impossible’, then he gets recommendations for movies of the action genre and the movies in which Tom Cruise is in the cast.
What makes Recommendations Engines work so well for OTT Platforms?
This section sheds light on why and how recommendations make OTT platforms more effective
Applications of recommendation engines in OTT
- OTT platform recommendation engine analyses the history and predicts future sensitivities of the user to engage them
- In fact, the Cloud-based recommenders even help OTTs/VODs to understand if the users are happy based on their requirements or not.
- It also produces knowledge graph-based recommendations that map to know what got the most user attention to drive better recommendations.
- It also uses reinforcement learning that can optimize the recommendation engine by spotting user preferences over time to make the recommendation funnel more relevant.
Content-Based Recommendation Engines
Here, based on what the customer searches, the recommendation system recommends all similar content to the user, e.g. when the user searches for a horror movie, it presents a list of best–rated horror movies.
Collaborative Recommendation Engines
Collaborative filtering works differently using a matrix-style formula. This recommendation is based on the users’ personalities. It recommends based on the search and preferences of a number of users who like the same kind of shows making the recommendations more valuable.
Hybrid Recommendation Systems
Hybrid recommendations use both the said types of systems to fetch much more improved suggestions to the user. Much accurate and broader spectrum of recommendations are produced by hybrid recommendation functions which are based on the behaviors, activities, and preferences of the users.
Data Procurement for Recommendation Engines
For the recommendation of results, data is the input; hence the acquisition of data is extremely important. Recommendation systems use data from user interaction, streaming content stored in databases, and user profiles.
This data is preprocessed, organized, cleaned for inaccuracies, and then presented to the user in an enriched form so that they have a usable recommendation listicle.
AI-based Recommendation Engines
AI-based recommendation systems work more intricately by analyzing catalogs, closed captions, and movie trailers too. Tools for comprehensive text analysis and context analysis are used and integrated with conventional machine learning models for better candidate generation.
AI-based recommendation systems work more intricately by analyzing catalogs, closed captions, and movie trailers too. Tools for comprehensive text analysis and context analysis are used and integrated with conventional machine learning models for better candidate generation
Custom-made Streaming Experience
Each customer has a different preference and interest. The recommendation engine can focus on each of these customers’ preferences equally. When new viewer opts for an OTT video platform, the recommendation tool provides them with what they are looking for and gives them a personalized OTT experience.
For instance, a customer who watches thriller series will get recommendations from those preferring comedy or action categories too.
Effortless Content Visibility
The recommendation system increases the visibility of the content by letting the viewers explore their content more accurately based on their preferences. E.g. when a customer watches a particular series on Netflix, the recommendation appears to watch this content on Amazon Prime in the customer’s language.
Hence the recommendation engine makes many OTT contents available easily, which would have been unexplored otherwise.
Extensive Video Analysis
Video content analysis (VCA) automatically studies videos by pulling out metadata from videos and finding video similarities more intricately. This is done by recommendation engines that also have deep learning capabilities to make the streaming experience more amusing to the viewers.
These modern recommendation engines use thumbnails, trailers, cast, etc. to detect similar videos.
Big Data Management
An OTT platform may need to use a lot of data involving various aspects like feedback, reviews, social media, online forums, etc. for appropriate recommendations. There are large datasets for computing patterns and trends associated with user behaviors, contexts, and products. This enormous data processing and also management system surely yield satisfactory recommendation results offering a better customer experience.
Extensive Video Analysis
Video content analysis (VCA) automatically studies videos by pulling out metadata from videos and finding video similarities more intricately. This is done by recommendation engines that have deep learning capabilities to make the streaming experience more amusing to the viewers. These modern recommendation engines also use thumbnails, trailers, cast, etc. to detect similar videos.
Big Data Management
Choosing the best OTT platforms may need to use a lot of data involving various aspects like feedback, reviews, social media, online forums, etc. for appropriate recommendations. There are large datasets for computing patterns and trends associated with user behaviors, contexts, and products. This enormous data processing and also management system surely yield satisfactory recommendation results offering a better customer experience.
Recap
So, that was a look at how OTT recommendation engines are helping OTT businesses predict consumer interests and turn them into responses to extend their scale. Without personalization, the content fails to grab its audience even if you have exclusive and unique content.
Machine Learning and also AI-driven recommendation systems are contributing to the OTT platform’s revenue and success big time. Netflix gets 80% of its streams through its recommender systems.
So if you want to replicate this success, look at a powerful and comprehensive recommendation engine to reap profits, also create user experience, and improve retention rates, translating it into customer acquisition.